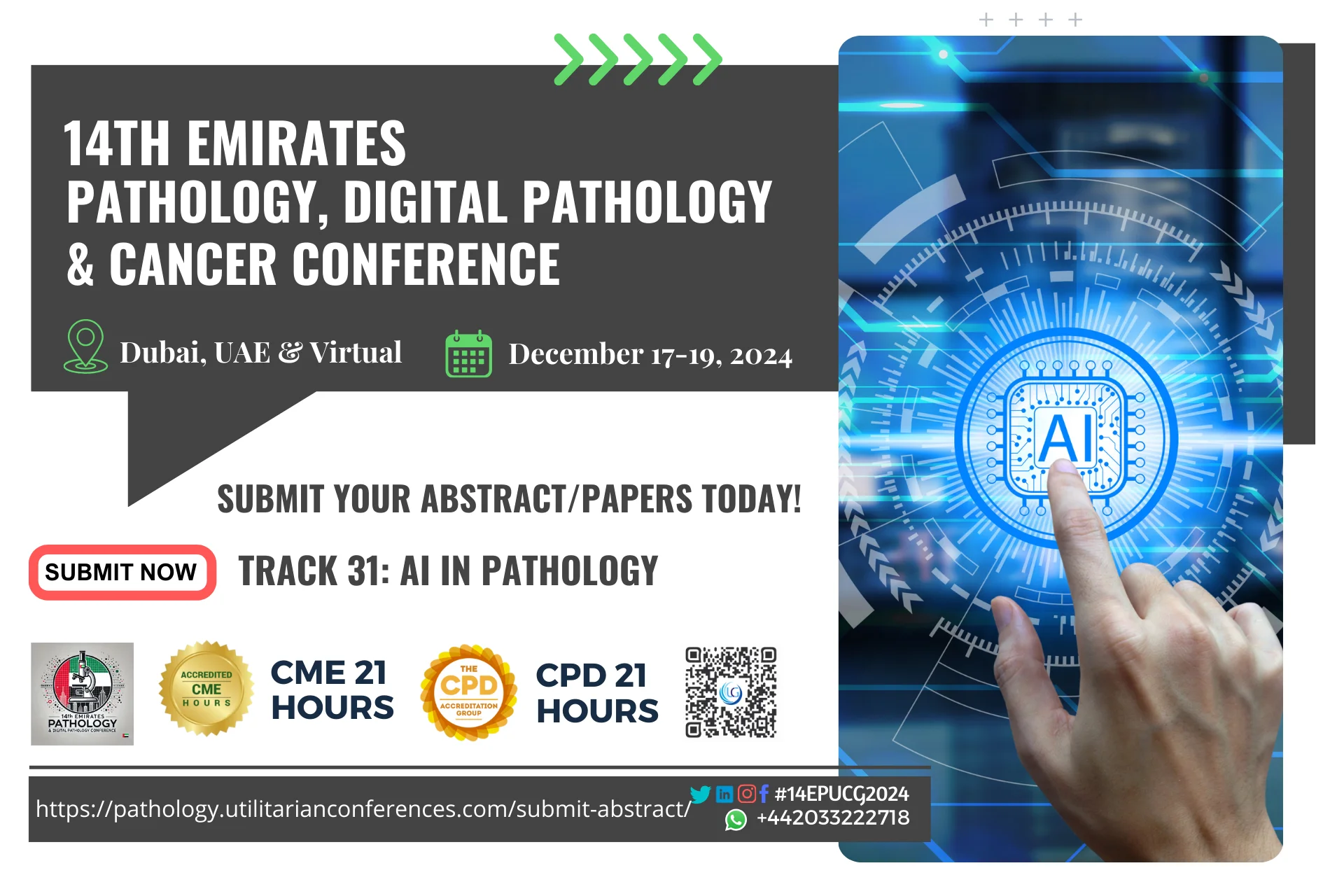
Call for Abstract/ Research Paper:
Sub Tracks: Pathology, pathology lab, pathology diagnosis, pathology...
Call for Abstract/ Research Paper:
Sub Tracks: Digital Pathology, Whole-Slide Imaging,...
Sub Topics: Digital Pathology, Machine Learning in Histopathology, Deep Learning Applications, Image Analysis and Interpretation, Automated Diagnosis, Predictive Analytics, AI-Driven Biomarker Discovery, Telepathology, Quality Control and Assurance, Integration with Electronic Health Records (EHR), AI in Cytopathology, Natural Language Processing in Pathology Reports, Computer-Aided Diagnosis (CAD), AI for Personalized Medicine, Ethics and Regulation of AI in Healthcare, Training and Validation of AI Models, Collaborative Robots (Cobots) in Pathology Labs, AI for Clinical Decision Support, Real-Time Pathology Insights, Future Trends in AI and Pathology
AI in Pathology refers to the application of artificial intelligence technologies, particularly machine learning and deep learning, to enhance the practice of pathology. By leveraging vast amounts of data and advanced algorithms, AI aims to improve diagnostic accuracy, efficiency, and overall patient outcomes in the field of pathology.
Key Applications of AI in Pathology:
Digital Pathology:
The transition from traditional glass slides to digital images allows for easier storage, sharing, and analysis. AI can analyze these digital slides for various diagnostic purposes.
Image Analysis and Interpretation:
AI algorithms can identify patterns in histopathological images, helping pathologists detect abnormalities such as tumors, inflammation, and other diseases more efficiently and accurately.
Machine Learning Models:
These models can be trained on large datasets of annotated pathology images to recognize specific features or classify tissue types. They can assist in diagnosing conditions like cancer, fibrosis, and infections.
Automated Diagnosis:
AI can aid in the initial diagnosis by automatically detecting and grading tumors, assessing the presence of specific biomarkers, and even providing differential diagnoses based on image analysis.
Predictive Analytics:
By analyzing historical patient data and outcomes, AI can help predict disease progression, treatment responses, and overall patient prognosis.
AI-Driven Biomarker Discovery:
AI can assist researchers in identifying new biomarkers associated with diseases, facilitating the development of targeted therapies and personalized medicine.
Quality Control and Assurance:
AI systems can monitor diagnostic processes to ensure consistency and accuracy, reducing the likelihood of errors in pathology labs.
Telepathology:
AI enhances telepathology by enabling remote diagnosis and consultation, making pathology services more accessible, especially in underserved areas.
Integration with Electronic Health Records (EHR):
AI can analyze data from EHRs to provide insights that help pathologists make informed decisions based on patient history, demographics, and previous test results.
AI in Cytopathology:
AI is increasingly used to analyze cytological samples (e.g., Pap smears, fine-needle aspirations), improving the detection of malignancies and other cellular abnormalities.
Natural Language Processing (NLP):
NLP technologies can be used to extract relevant information from pathology reports and medical literature, helping pathologists stay updated with current research and guidelines.
Computer-Aided Diagnosis (CAD):
CAD systems support pathologists by highlighting areas of interest in pathology slides, which can increase diagnostic efficiency and accuracy.
Collaborative Robots (Cobots):
These robots can assist pathologists in routine tasks, such as slide preparation and imaging, freeing up time for more complex analyses.
Ethics and Regulation:
The implementation of AI in pathology raises ethical considerations regarding data privacy, algorithmic bias, and the need for regulatory standards to ensure safety and efficacy.
Training and Validation:
Ongoing research is required to validate AI algorithms using diverse datasets to ensure generalizability and accuracy across different populations and pathology practices.
Challenges and Considerations:
Data Quality and Annotation: High-quality, annotated datasets are essential for training accurate AI models.
Integration into Clinical Practice: Ensuring that AI tools are seamlessly integrated into existing workflows and systems in pathology labs.
Interpretability: Understanding how AI models make decisions is crucial for gaining pathologists' trust and ensuring clinical utility.
Continuous Learning: AI models need to adapt and improve over time as new data and techniques emerge.
Conclusion:
AI in Pathology holds great promise for transforming the field by improving diagnostic accuracy, efficiency, and patient care. As technology advances, continued collaboration between pathologists, data scientists, and software engineers will be essential to realize the full potential of AI in pathology.